In part 1, we explored risk model issues and the need for skepticism. Now, let's focus on adapting to these challenges.
Validation
Let’s start with the models themselves.
Even if you aren’t using a formal model, there are a lot of things you probably know today. You likely have at least a working sense of which information assets support critical, revenue-generating business. You can augment this knowledge and may discover some new things by doing a revenue-process map linked into a data flow — a practice we’ve found invaluable in optimizing security efforts.
And you don’t have to wait to complete one of those to sharpen your model. Look at incident history to your critical processes and convert those to event trees to get relatively objective (albeit history-based) probabilities. It’s a defensible start as you gather more intel.
Speaking of probabilities, a mistake I see too often are security teams overly focused on likelihood while normalizing or ignoring impact. Unless your IT shop has done a world-class job optimizing your data footprint, it’s not likely that these two are independent. The business does need to provide their input on impact, and that can be a key input as you do your business process mapping. If you have little impact data today, a half-day workshop with the right business executives at your next off-site can get you and your team a lot of insight on how to weigh impact, or map existing impact scales to assets.
In previous posts we’ve stressed the merits of shifting to more quantitative modeling. It’s easy to hide behind fuzzy data with qualitative models, which makes them a safety blanket when being grilled in front of your board. However, I am witnessing many boards starting to see through that, and pushing for risk reporting at parity with other business units. That parity is often anchored in dollars and cents. I know it’s not easy, but it does simplify the discussion around risk, what it means, how big it is, and how it compares with other risks and risk mitigation costs. Start small, but if you aren’t starting to move in this direction, you will soon be behind.
Finally, stress-test assumptions in your model and root out bias. Run simulations through the models you have built and used to see how they perform under certain circumstances. Specifically look at the impacts of major changes to a few variables. Stress-testing will highlight potential blind spots as well as ways to improve the model.
Get soft data too
I can’t stress this enough: Front line employees’ understanding of security risk should not be underestimated. That doesn’t just mean that they follow the rules, but they tend to have a sense of where weaknesses are. Just as some of the best CEOs walk the floor of their companies to see what is really going on, so should the best CISOs (and their risk teams).
Gather intel on what weak points there are and what keeps them up at night (at least Business Line execs). While you are at it, use the opportunity to see how security can be more helpful or how security is creating perceived barriers that you can remove. These win-win partnerships are invaluable.
I have to admit that I have seen mixed results in having security or risk liaisons in different business groups. I used to be a strong proponent, but have seen that those liaisons drift to the needs of the business line over time i.e. making things easier, not more secure. The real bounty comes from incorporating risk management into the day-to-day jobs of all employees and bubbling it up (see this HBR highlight of Hydro One). Tight integration is fostered via education, tone-at-the-top, and incentives to appropriately owning and managing risk on the front lines.
Greenspan used to check on underwear sales at department stores as a way to see how well economic models were likely to perform, because underwear sales were the first thing to plummet when the economy was weak (clothing you can’t see!). Soft data is a vital way to validate your risk models…to get “out of the box” and into the real world.
Governance
I’m sure you’ve heard that you can have the best risk models and intel in the world, but without good risk governance it won’t go far. The inverse is true as well: even with immature risk models and intel, having good risk governance will go a long way. Risk models are just tools, and how you use them and the arsenal of other tools at your disposal is key to the ultimate objective: reducing risk to tolerable levels.
Put in place a risk operating model that can act on what we know. Existing risk assessment results, vulnerability or pen testing, and incidents all provide good data to point to areas that likely need improvement. The risk model can help optimize and prioritize, but the real key is real progress. I have been in so many organizations with world class risk governance at the heart of business operations, but almost non-existent in the back-office / IT. Get the foundational pieces of GRC in place, the right voices at the table to make priority decisions, and the right sponsorship to see it through. You can focus on improving the data these governance boards get over time. But move forward with governance structures, and you’ll make an impact.
Continuity Planning
A prominent CISO told me early in my career that the first two areas he gets right-sized when he walks into a company are vulnerability management and incident response. Though there may be qualified responses to that, there is a lot of wisdom there too. The bad guys are constantly looking for your vulnerabilities and when something inevitably occurs, you need to be ready.
Given the surprises and shocks to business that have occurred over the last couple of decades, business continuity is taking on a more prominent role, and never more than with this pandemic. And I hope it lasts (the business continuity focus….NOT the pandemic!). So aside from strengthening risk models and getting your risk management operating model in good shape, my final recommendation is to beef up your business continuity and incident response programs.
Let’s use COVID-19 as a lesson on how to do that. No one could have predicted we’d be in such a position. Even Bill Gates, warning about a pandemic for years, didn’t think we’d get to this point. But here we are. So what does that mean for the continuity plans that were half baked and got beaten up during this whole episode?
It means we need to focus these plans not on causes, but on effects. We can’t predict everything that can or will happen…there are an infinite number of possibilities in fact. But we can focus on the results, and what options we can put in place to secure those. Those results are generally losses. Loss of resources, loss of people, loss of facilities, loss of customers. If you have a sudden loss of 50% of your workforce (let’s say this virus was even more contagious, or, heaven forbid, deadlier), what do you do to survive?
Develop a survival plan that might include cross training for your most critical services. Think about what services are critical, what losses could occur (despite how they occur), and what needs to be put in place to mitigate for potential losses. This kind of planning complements your risk models and program, unifying them into one integrated approach that increases resilience across the enterprise.
Conclusion
Risk models, though informative, have their limitations. I’m not ready to throw them out, but they need to be tempered with healthy skepticism and stress-testing. Security is a tough area, and, while silver bullets would be nice, they just don’t exist for this space. We need the people and processes along with the tools to do this right. Good models, validation, governance, continuity planning, coupled with a healthy dose of continuous measurement and improvement will put you in good stead with your customers and your Board.
Author
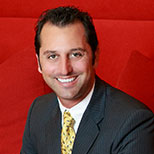